Understand Fatigue Performance of Metal Additively Manufactured Parts with Data-driven Modeling
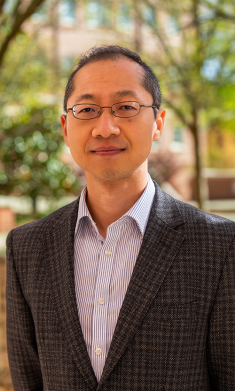
Jia (Peter) Liu, PhD
Auburn University
Abstract: Additive manufacturing (AM) offers unprecedented flexibility in freeform fabrication using a layer-wise strategy for product innovation, mass customization and sustainable production. With a laser scanning metal powder layer by layer, laser beam powder bed fusion (L-PBF) provides high geometry accuracy and strength for different applications. Though L-PBF fabricated parts have comparable static mechanical properties with their counterparts from wrought materials, their dynamic properties under cyclic loading are inferior due to their nonhomogeneous microstructure from inconstant process
characteristics. Their fatigue performance has more uncertainty and larger variability than their wrought counterparts do. Since fatigue failure has historically caused the majority of engineering failure incidents, the uncertainty in the fatigue performance of L-PBF parts thwarts the adoption of such innovative technology in fatigue-critical applications. In this talk, I will introduce our work in data-driven modeling to advance the understanding of microstructural traits contributing to fatigue fracture of L-PBF parts and entail non-destructive fatigue life prediction. Fatigue failure is usually attributed to crack initiation and propagation by fracture mechanics in microstructural traits. Two dominant microstructural traits of L-PBF parts (volumetric defects and surface roughness) that induce crack initiation and fatigue fracture are characterized and modeled based on physics knowledge and experimental results. These models not only utilize physics knowledge in L-PBF, but also leverage the power of data-driven methods to address the issues of complex physics and data sparsity to advance non-destructive fatigue life prediction for L-PBF parts and their potential adoption to more fatigue-critical applications.
Biography: Dr. Jia (Peter) Liu is an Assistant Professor in the Department of Industrial and Systems Engineering at Auburn University. He graduated from Virginia Tech with a Ph.D. in Industrial and Systems Engineering and an M.S. in Statistics in 2017. He also received a B.S. and an M.S. in Electrical Engineering from Zhejiang University, China, in 2005 and 2007, respectively. He focuses on interpretable data-driven modeling for complex manufacturing processes with heterogeneous sensor data to achieve online process monitoring, product quality prediction, and control. He has published over 40 journal and conference papers. His research contribution has been featured in IISE magazine. He has been honored with several awards, including the 2023 NSF Career Award, the 2023 Ginn Achievement Faculty Fellow at Auburn University, and the 2020 Early Career Development Grant at Auburn University.